DABI: Evaluation of Data Augmentation Methods Using Downsampling in Bilateral Control-Based Imitation Learning with Images
Osaka University
Masato Kobayashi*, Thanpimon Buamanee*, Yuki Uranishi
* Co-first authors equally contributed to this work.
Abstract:
Autonomous robot manipulation is a complex and continuously evolving robotics field. This paper focuses on data augmentation methods in imitation learning. Imitation learning consists of three stages: data collection from experts, learning model, and execution. However, collecting expert data requires manual effort and is time-consuming. Additionally, as sensors have different data acquisition intervals, preprocessing such as downsampling to match the lowest frequency is necessary. Downsampling enables data augmentation and also contributes to the stabilization of robot operations. In light of this background, this paper proposes the Data Augmentation Method for Bilateral Control-Based Imitation Learning with Images, called “DABI”. DABI collects robot joint angles, velocities, and torques at 1000 Hz, and uses images from gripper and environmental cameras captured at 100 Hz as the basis for data augmentation. This enables a tenfold increase in data. In this paper, we collected just 5 expert demonstration datasets. We trained the bilateral control Bi-ACT model with the unaltered dataset and two augmentation methods for comparative experiments and conducted real-world experiments. The results confirmed a significant improvement in success rates, thereby proving the effectiveness of DABI.
DABI
Put-in-Drawer Task using Proposed Method “DABI” (autonomous and real-time 1x)
DABI
Experiments
Experimental Environments
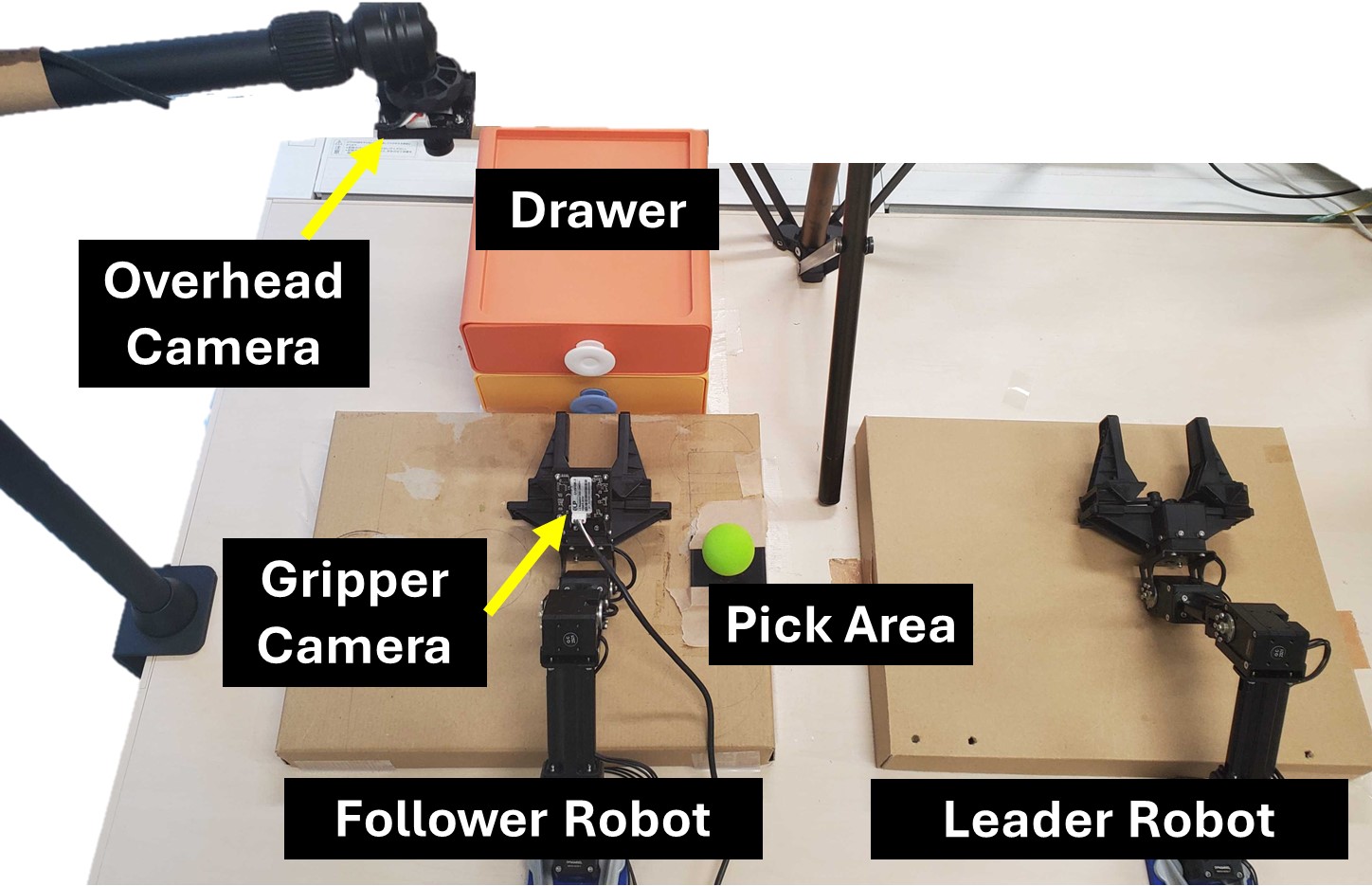
Experimental Environments
Data Collection using Bilateral Control
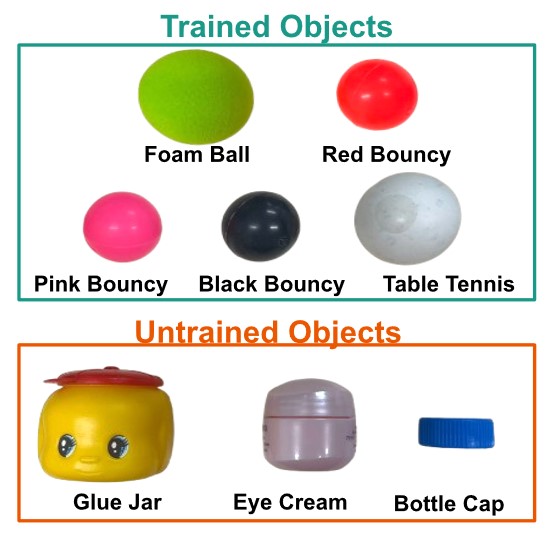
Objects
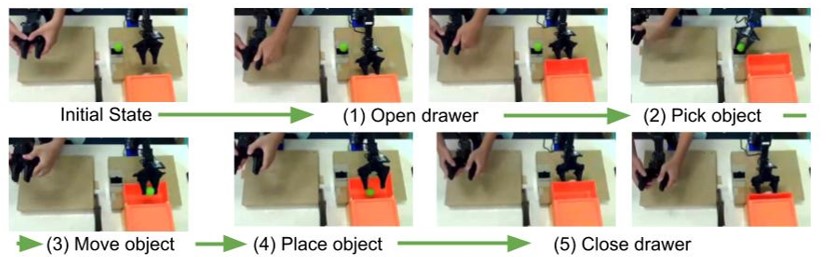
Data Collection
DABI
The Put-in-Drawer task requires five steps: opening the drawer, picking up the object, moving the object, placing the object, and closing the drawer. In the Put-in-Drawer task, we collected a total of 5 demonstration data, gathering one demonstration for each of the five different objects by bilateral control. Two types of images(100Hz). Robot data of joint position, velocity, torque (1000Hz).
Model (Bi-ACT: Bilateral Control-Based Imitation Learning via Action Chunking with Transformer)
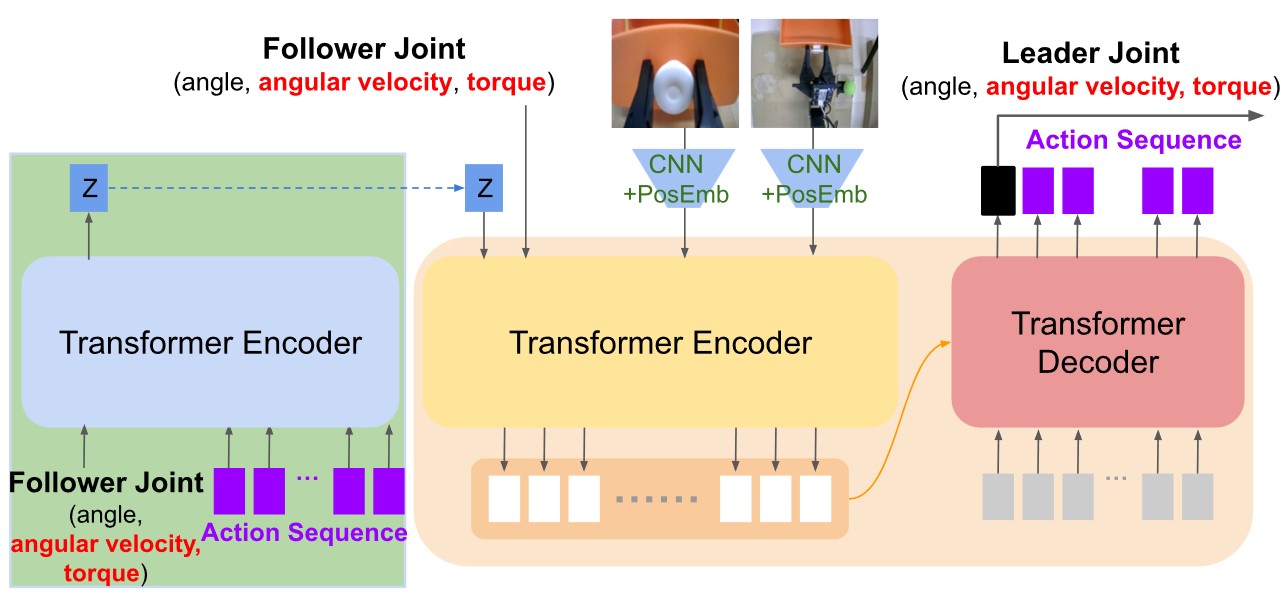
Bi-ACT Model
Put-in-Drawer Task using Proposed Method “DABI” (autonomous and real-time 1x)
DABI
Citation
@misc{kobayashi2024dabi,
title={DABI: Evaluation of Data Augmentation Methods Using Downsampling in Bilateral Control-Based Imitation Learning with Images},
author={Masato Kobayashi and Thanpimon Buamanee and Yuki Uranishi},
year={2024},
eprint={2410.04370},
archivePrefix={arXiv},
primaryClass={cs.RO}
}
Contact
Masato Kobayashi (Assistant Professor, Osaka University, Japan)
- X (Twitter)
- English : https://twitter.com/MeRTcookingEN
- Japanese : https://twitter.com/MeRTcooking
- Linkedin https://www.linkedin.com/in/kobayashi-masato-robot/